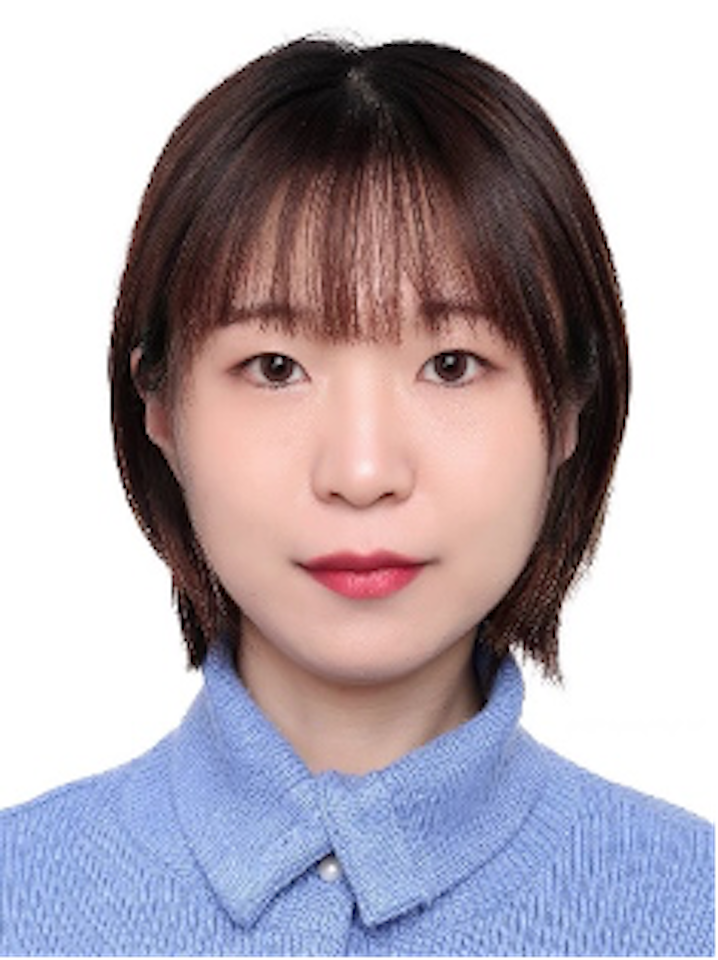 Yuhan CHEN |
Yuhan CHEN
Department of Mathematics,
Graduate School of Science, Kobe University |
Division : Applied Mathematics
Assistant Professor |
Building B, Room 327

Personal Website
|
Research Field :
Deep Learning for Modelling and Simulations in Physics |
|
Research Summary :
Data-driven modeling methods, especially those based on deep learning, have attracted much attention in recent years.
Well-known models that combine deep learning and physics include Hamiltonian neural networks and Physics Informed Neural Networks.
My research combines deep learning and geometric mechanics theory to construct models that preserve physical properties and to analyze these properties theoretically.
At the same time, I aim to reduce errors and improve the accuracy of physical simulations by simplifying more complex deep physical models.
|
|
Primary Publications : |
-
Y. Chen, T. Matsubara and T. Yaguchi, “Super resolution of numerical solutions of nonlinear elliptic equations by
DeepONet,” International Symposium on Nonlinear Theory and Its Applications(NOLTA2023), Catania, Sep 2023.
-
Y. Chen, B. Xu, T. Matsubara and T. Yaguchi, “Geometric Integrators for Neural Symplectic Forms,” International Congress onIndustrial and Applied Mathematics(ICIAM2023,Minisymposium), Tokyo, Aug 2023.
-
Y. Chen, B. Xu, T. Matsubara and T. Yaguchi, “Variational Principle and Variational Integrators for Neural Symplectic Forms,” International Conference on Machine Learning (ICML2023,workshop), Honolulu, Jul 2023.
-
Y. Chen, T. Matsubara and T. Yaguchi, “Variational Integrator for Hamiltonian Neural Networks,” International Symposium on Nonlinear Theory and Its Applications (NOLTA2022), Student paper award, Virtual, Dec 2022.
-
Y. Chen, T. Matsubara and T. Yaguchi, “Neural symplectic form and coordinate-free learning of Hamiltonian dynamics,” International Conference on Scientific Computation and Differential Equations (SciCADE), Iceland, Jul 2022.
-
Y. Chen, T. Matsubara and T. Yaguchi, “KAM Theory Meets Statistical Learning Theory: Hamiltonian Neural Networks with Non-Zero Training Loss,” Association for the Advancement of Artificial Intelligence (AAAI2022), Oral Presentation (Oral acceptance rate 4.6%), Feb 26, 2022.
-
Y. Chen, T. Matsubara and T. Yaguchi, “Neural Symplectic Form: Learning Hamiltonian Equations on General Coordinate Systems,” Conference on Neural Information Processing Systems (NeurIPS2021), Splotlight Presentation (Splotlight acceptance rate 3%), Virtual, Dec 2021.
-
Y. Chen, H. Sano, M. Wakaiki and T. Yaguchi, “Secret Communication Systems Using Chaotic Wave Equations with Neural Network Boundary Conditions,” Entropy, 23, 904. (2021)
|
|
|